Tissue cytometry
![]() | A major contributor to this article appears to have a close connection with its subject. (December 2024) |
Tissue image cytometry or tissue cytometry[1] is a method of digital histopathology and combines classical digital pathology (glass slides scanning and virtual slide generation) and computational pathology (digital analysis) into one integrated approach with solutions for all kinds of diseases, tissue and cell types as well as molecular markers and corresponding staining methods to visualize these markers. Tissue cytometry uses virtual slides as they can be generated by multiple, commercially available slide scanners, as well as dedicated image analysis software – preferentially including machine and deep learning algorithms.[2][3][4] Tissue cytometry enables cellular analysis within thick tissues, retaining morphological and contextual information, including spatial information on defined cellular subpopulations.[1][5]

In this process, a tissue sample, either formalin-fixed paraffin-embedded (FFPE) or frozen tissue section, also referred to as “cryocut”, is labelled with either immunohistochemistry[6](IHC) or immunofluorescent markers, scanned with high-throughput slide scanners and the data gathered from virtual slides is processed and analyzed using software that is able to identify individual cells in tissue context automatically and distinguish between nucleus and cytoplasm for each cell.[2][4] Additional algorithms can identify cellular membranes, subcellular structures (like cytoskeletal fibers, vacuoles, nucleoli) and/or multicellular tissue structures (glands, glomeruli, epidermis, or tumor foci).[7]Fluorescence Activated Cell Sorting (FACS) is a method of analysis that measures fluorescence signals on single cells, where the signal comes from antibody-mediated staining techniques and phenotypes detected by flow cytometry.[8] The major limitation of flow cytometry is that it can only be applied – as the name suggest – to cells in solution. Although methods of “solubilizing” solid tissue exist, any such processing irrevocably destroys the tissue architecture and any spatial context. Hence, tissue cytometry complements the use of flow cytometry and fluorescence microscope[9] in basic research, clinical practice, and clinical trials by providing FACS-like analyses on solid tissue sections (as well as adherent cell cultures) in situ. The advantage of tissue cytometry against flow cytometry is that tissue cytometry does not require the cells to be suspended in fluid, aiding in maintaining the integrity of the tissue structure, morphology, and contextual information, further assisting in precise and accurate contextual analysis that are not possible in flow cytometry.
History
[edit]Immunohistochemistry is used in clinical practice, where tissue biopsies from every potential cancer patient are collected, fixed in formalin and embedded on paraffin. These tissue sections are serially cut in a microtome to provide thin sections, representing the diagnostic material for clinical diagnoses.[6] Once stained initially with hematoxylin and eosin stain to detect cancer cells. Multiple marker staining is performed for proliferation, lineage, prognostic and oncogenic targets. Pathologists used optical microscope for the evaluation through the objective lenses and conclude the diagnosis by scoring the staining in percentage or as positive/negative. Visual evaluation provides a subjective diagnosis and plan of treatment. The advent of digital pathology marked a significant leap forward in the field of pathology. By converting glass slides into digital images, it revolutionized how pathologists interacted with tissue specimens. However, the initial phase of digital pathology primarily focused on image viewing and sharing. While this enabled remote consultations and facilitated image archiving, it did not fundamentally alter the core process of pathology: the manual interpretation of tissue morphology by human experts.
A more robust and automated system was designed to perform flow cytometry-like analyses on immunostained cells in a fixed tissue and termed tissue cytometry.[10][11] The technique was introduced in the 1990s based on patents by Steiner and Ecker (CEO/founder TissueGnostics),[12] describing a procedure for “Cytometric Analysis of Diverse Cell Populations in Tissue Sections or Cell Culture Visualized Through Fluorescence Dyes and/or Chromogens". Tissue cytometry emerged as a transformative extension of digital pathology, promising to bridge the gap between image-based analysis and quantitative, data-driven insights. This technology leverages advanced imaging techniques, computational power, and artificial intelligence (AI) to extract meaningful information from tissue slides. Unlike its predecessor, tissue cytometry goes beyond mere image visualization, delving into the complex spatial and molecular landscape of tissue specimens. At its core, tissue cytometry enables the automated and quantitative analysis of cellular and tissue features. By employing sophisticated algorithms and machine learning models, it can accurately segment nuclei, identify cell types, and quantify protein expression levels within the tissue context. This level of granularity and precision was previously unattainable through traditional microscopic examination.
Additional patents were filed in the early 21st century by Hernani et al. to perform virtual flow cytometry on immunostained tissue.[13] The latter's basics were derived from the procedure presented in 1982 by Gillete et al., describing the qualitative analysis of spectral mixtures by using factor analysis in conjunction with a spectral reference library.[14] Following this study, Zhou R et al. published a method to quantify prostate-specific acid phosphatase (PSAP) in histologic sections of prostate tumor with the peroxidase-antiperoxidase (PAP) complex technique using diaminobenzidine (DAB) as a substrate.[15]
The integration of AI and machine learning has been instrumental in the development of tissue cytometry. These technologies empower the system to learn from vast datasets of annotated tissue images, enabling it to recognize patterns and make accurate predictions. For instance, AI-driven algorithms can be trained to identify specific cell types, detect subtle morphological changes associated with disease, or quantify the density of immune cells within a tumor microenvironment.
Moreover, AI-powered nuclear segmentation is a critical component of tissue cytometry. By precisely delineating individual nuclei, researchers can extract valuable information about nuclear size, shape, and texture, which can be correlated with various pathological conditions. Similarly, tissue segmentation algorithms enable the identification of different tissue compartments, such as tumor, stroma, and immune infiltrate, facilitating the analysis of spatial relationships between cellular components.
The implications of tissue cytometry for pathology are profound. By providing quantitative and reproducible data, this technology has the potential to streamline the diagnostic and prognostic processes. For example, tissue cytometry can assist pathologists in identifying subtle biomarkers that may be missed during manual examination, leading to earlier and more accurate diagnoses. Furthermore, by quantifying tumor burden and immune infiltration, tissue cytometry can help predict patient outcomes and inform treatment decisions.
Beyond diagnostics, tissue cytometry opens up new avenues for drug development and clinical research. By enabling precise phenotyping of tumor cells and immune microenvironments, it can facilitate the identification of potential therapeutic targets and the evaluation of drug efficacy. Additionally, tissue cytometry can be used to study the mechanisms of disease progression and resistance, paving the way for the development of novel treatment strategies.
Tissue cytometry Environment/ Tissue Cytometers
[edit]Modern tissue cytometers can analyze many thousands of cells within the tissue sample and/or in suspension, in "real time" and, if configured as cell sorters, can actively distinguish particles with specified grey value.
A tissue cytometer has 3 main components- A high-throughput scanner to acquire the high-quality virtual image of immunohistochemical and/or fluorescent marker labelled tissue sections on glass slides, a signal detector to detect the brightfield or fluorescent signals in Red Green Blue (RGB) format and, a computer with developed algorithm for the analysis and interpreting of the signals in grey values.
Applications of Tissue cytometry
[edit]
Tumor Microenvironment: Tissue cytometry is heavily used in research to characterize the tumor microenvironment including e.g. identification of the immune landscape or tumor-vascularization, within IHC/IF-processed tissue sections. One reason is that by using this technology the complex tissue architecture stays intact and therefore also spatial relationships between cellular phenotypes and/or multicellular structures can be analyzed.[16]
By utilizing tissue cytometry multiple research groups were able to demonstrate the impact of various immune cell subpopulations (CD4, CD68, CD8, CD20, Foxp3, PD1) on patient survival in different cancer types (e.g. breast cancer, colon cancer, gastric cancer, melanoma, non-small cell lung cancer).[16] Since in cancer therapy a novel treatment strategy is targeting immune checkpoints (molecules that inhibit the antitumoral immune reaction), the insights gained by tissue cytometry may help to find new target molecules/biomarkers as well as to determine the best treatment strategy for patients.[16]
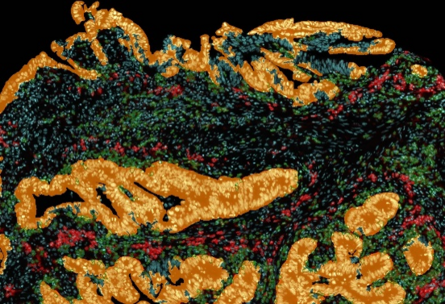
Immunology: Immune cell context is important for delineating the etymology of inflammatory diseases, which often result from impaired function of adaptive and/or innate immune cells. Tissue cytometry is useful for detecting and localizing specific cells, especially heterogeneous populations, within their native tissue environment and identifying the cues behind the disease.[17] For example, it was used to investigate IgG4-related diseases: one paper reports about fibrosing mediastinitis being driven by CD4+ CTLs rather than Th2 cells where infiltration of CD4+ CTLs was illustrated by tissue cytometry.[18] Follow-up studies investigated how follicular T cells influence B-cell class-switching events in IgG4-related disease and Kimura disease – researchers found a correlation between AICDA+CD19+ B cells and IgG4 expression using tissue cytometry.[19]
Mesenchymal Stem Cells Characterization: Mesenchymal stem cells (MSCs) are multipotent cells that have the capacity differentiate into several sub-types such as bone, cartilage, muscle, developing teeth and fat tissue which has clinical importance for regenerative medicine.[20] However, although there are defined minimal phenotypic criteria, MSCs due to their heterogeneous nature need to be further characterized regarding their distinct biomarkers.[21] Tissue cytometry promisingly assists to describe the biomarkers of quiescent MCSs and furthermore characterize the effect of hyaluronan on this population.[22] Tissue cytometry can also used to investigate MSCs interaction with glioblastoma: to characterize cell fusion, extracellular vesicle transfer and intercellular communications.[23] Additionally, tissue cytometry is utilized to image the murine hippocampus and visualize M1/M2 microglia in mice with MSCs transplantation as a model for Alzheimer’s disease.[24]

COVID-19: COVID-19 pandemic required various tools to outline the disease progression and severity. Using tissue cytometry, researchers reported about interplay of immune cells and SARS-CoV-2 virus and its effect on disease: for instance, one study showed that CD4+ cytotoxic T cells expanded significantly in the lungs in severe COVID-19.[25] Another finding illustrates loss of germinal centers in lymph nodes and spleens in acute COVID-19, which was shown by multi-color immunofluorescence cytometry.[26]
Neuroscience: Tracking neurodevelopmental processes is an active field of research in neuroscience. Quantitative tissue analysis is widely employed in the field to determine the role of different stimuli in the nervous system.[27][28][29][30] A research group reported about the effect of the magnetic field on neural differentiation of pluripotent stem cells, where the phenotypic effects were observed using tissue cytometry.[27] Another application of tissue cytometry in neuroscience was shown in a study designed to evaluate the effect of stress on hypothalamic neurons.[28]
References
[edit]- ^ a b "Image Cytometry Technology and Tissue Analysis". News-Medical.net. 2018-09-04. Retrieved 2022-07-07.
- ^ a b Cualing, Hernani D.; Zhong, Eric; Moscinski, Lynn (2006). ""Virtual flow cytometry" of immunostained lymphocytes on microscopic tissue slides:iHCFlow™ tissue cytometry". Cytometry Part B. 72B (1): 63–76. doi:10.1002/cyto.b.20148. ISSN 1552-4949. PMID 17133379. S2CID 36237785.
- ^ "EuroLab Tech June 2021". content.yudu.com.
- ^ a b "Home". tissuegnostics.com. Retrieved 2021-08-04.
- ^ Ferkowicz, Michael J.; Winfree, Seth; Sabo, Angela R.; Kamocka, Malgorzata M.; Khochare, Suraj; Barwinska, Daria; Eadon, Michael T.; Cheng, Ying-Hua; Phillips, Carrie L.; Sutton, Timothy A.; Kelly, Katherine J. (2021). "Large-scale, three-dimensional tissue cytometry of the human kidney: a complete and accessible pipeline". Laboratory Investigation. 101 (5): 661–676. doi:10.1038/s41374-020-00518-w. ISSN 1530-0307. PMC 8363780. PMID 33408350.
- ^ a b Duraiyan, Jeyapradha; Govindarajan, Rajeshwar; Kaliyappan, Karunakaran; Palanisamy, Murugesan (August 2012). "Applications of immunohistochemistry". Journal of Pharmacy & Bioallied Sciences. 4 (Suppl 2): S307–S309. doi:10.4103/0975-7406.100281. ISSN 0976-4879. PMC 3467869. PMID 23066277.
- ^ Imaging Modalities for Biological and Preclinical Research: A Compendium Volume One. IOP Publishing. 2021. pp. I.2.h-1-I.2.h-10.
- ^ McKinnon, Katherine M. (2018-02-21). "Flow Cytometry: An Overview". Current Protocols in Immunology. 120: 5.1.1–5.1.11. doi:10.1002/cpim.40. ISSN 1934-3671. PMC 5939936. PMID 29512141.
- ^ Sanderson, Michael J.; Smith, Ian; Parker, Ian; Bootman, Martin D. (2014-10-01). "Fluorescence Microscopy". Cold Spring Harbor Protocols. 2014 (10): pdb.top071795. doi:10.1101/pdb.top071795. ISSN 1940-3402. PMC 4711767. PMID 25275114.
- ^ "Tissue Cytometry | Flow Cytometry | What's the Difference?". tissuegnostics.com. Retrieved 2022-07-04.
- ^ El-Achkar, Tarek M.; Winfree, Seth; Talukder, Niloy; Barwinska, Daria; Ferkowicz, Michael J.; Al Hasan, Mohammad (2022). "Tissue Cytometry With Machine Learning in Kidney: From Small Specimens to Big Data". Frontiers in Physiology. 13: 832457. doi:10.3389/fphys.2022.832457. ISSN 1664-042X. PMC 8931540. PMID 35309077.
- ^ USPTO.report. "Method and system for analyzing cells Patent Application". USPTO.report. Retrieved 2022-07-07.
- ^ Cualing, Hernani D.; Zhong, Eric; Moscinski, Lynn (2007-01-15). ""Virtual flow cytometry" of immunostained lymphocytes on microscopic tissue slides: iHCFlow tissue cytometry". Cytometry Part B. 72 (1): 63–76. doi:10.1002/cyto.b.20148. ISSN 1552-4949. PMID 17133379. S2CID 36237785.
- ^ Gillette, Paul C.; Lando, Jerome B.; Koenig, Jack L. (1983-04-01). "Factor analysis for separation of pure component spectra from mixture spectra". Analytical Chemistry. 55 (4): 630–633. doi:10.1021/ac00255a011. ISSN 0003-2700.
- ^ Zhou, R.; Parker, D. L.; Hammond, E. H. (1992). "Quantitative peroxidase-antiperoxidase complex-substrate mass determination in tissue sections by a dual wavelength method". Analytical and Quantitative Cytology and Histology. 14 (2): 73–80. PMID 1590900.
- ^ a b c Mungenast, Felicitas; Fernando, Achala; Nica, Robert; Boghiu, Bogdan; Lungu, Bianca; Batra, Jyotsna; Ecker, Rupert C. (2021-04-07). "Next-Generation Digital Histopathology of the Tumor Microenvironment". Genes. 12 (4): 538. doi:10.3390/genes12040538. ISSN 2073-4425. PMC 8068063. PMID 33917241.
- ^ Gerner, Michael Y.; Kastenmuller, Wolfgang; Ifrim, Ina; Kabat, Juraj; Germain, Ronald N. (2012-08-24). "Histo-cytometry: a method for highly multiplex quantitative tissue imaging analysis applied to dendritic cell subset microanatomy in lymph nodes". Immunity. 37 (2): 364–376. doi:10.1016/j.immuni.2012.07.011. ISSN 1097-4180. PMC 3514885. PMID 22863836.
- ^ Allard-Chamard, Hugues; Alsufyani, Faisal; Kaneko, Naoki; Xing, Kelly; Perugino, Cory; Mahajan, Vinay S.; Wheat, Joseph L.; Deepe, George S.; Loyd, James; Pillai, Shiv (2021-02-01). "CD4+CTLs in Fibrosing Mediastinitis Linked to Histoplasma capsulatum". The Journal of Immunology. 206 (3): 524–530. doi:10.4049/jimmunol.2000433. ISSN 0022-1767. PMC 7978153. PMID 33328214.
- ^ Munemura, Ryusuke; Maehara, Takashi; Murakami, Yuka; Koga, Risako; Aoyagi, Ryuichi; Kaneko, Naoki; Doi, Atsushi; Perugino, Cory A.; Della-Torre, Emanuel; Saeki, Takako; Sato, Yasuharu; Yamamoto, Hidetaka; Kiyoshima, Tamotsu; Stone, John H.; Pillai, Shiv (August 2022). "Distinct disease-specific Tfh cell populations in 2 different fibrotic diseases: IgG4-related disease and Kimura disease". The Journal of Allergy and Clinical Immunology. 150 (2): 440–455.e17. doi:10.1016/j.jaci.2022.03.034. ISSN 1097-6825. PMC 10369367. PMID 35568079.
- ^ Ding, Dah-Ching; Shyu, Woei-Cherng; Lin, Shinn-Zong (2011). "Mesenchymal stem cells". Cell Transplantation. 20 (1): 5–14. doi:10.3727/096368910X. ISSN 1555-3892. PMID 21396235. S2CID 7868466.
- ^ Baer, Patrick C. (2014-07-26). "Adipose-derived mesenchymal stromal/stem cells: An update on their phenotype in vivo and in vitro". World Journal of Stem Cells. 6 (3): 256–265. doi:10.4252/wjsc.v6.i3.256. ISSN 1948-0210. PMC 4131268. PMID 25126376.
- ^ Wong, Tzyy Yue; Chang, Chiung-Hsin; Yu, Chen-Hsiang; Huang, Lynn L. H. (June 2017). "Hyaluronan keeps mesenchymal stem cells quiescent and maintains the differentiation potential over time". Aging Cell. 16 (3): 451–460. doi:10.1111/acel.12567. ISSN 1474-9726. PMC 5418204. PMID 28474484.
- ^ Pillat, Micheli Mainardi; Oliveira-Giacomelli, Ágatha; das Neves Oliveira, Mona; Andrejew, Roberta; Turrini, Natalia; Baranova, Juliana; Lah Turnšek, Tamara; Ulrich, Henning (February 2021). "Mesenchymal stem cell-glioblastoma interactions mediated via kinin receptors unveiled by cytometry". Cytometry. Part A. 99 (2): 152–163. doi:10.1002/cyto.a.24299. ISSN 1552-4930. PMID 33438373. S2CID 231594242.
- ^ Campos, Henrique C.; Ribeiro, Deidiane Elisa; Hashiguchi, Debora; Hukuda, Deborah Y.; Gimenes, Christiane; Romariz, Simone A. A.; Ye, Qing; Tang, Yong; Ulrich, Henning; Longo, Beatriz Monteiro (February 2022). "Distinct Effects of the Hippocampal Transplantation of Neural and Mesenchymal Stem Cells in a Transgenic Model of Alzheimer's Disease". Stem Cell Reviews and Reports. 18 (2): 781–791. doi:10.1007/s12015-021-10321-9. ISSN 2629-3277. PMID 34997526. S2CID 255446340.
- ^ Kaneko, Naoki; Boucau, Julie; Kuo, Hsiao-Hsuan; Perugino, Cory; Mahajan, Vinay S.; Farmer, Jocelyn R.; Liu, Hang; Diefenbach, Thomas J.; Piechocka-Trocha, Alicja; Lefteri, Kristina; Waring, Michael T.; Premo, Katherine R.; Walker, Bruce D.; Li, Jonathan Z.; Gaiha, Gaurav (April 2022). "Temporal changes in T cell subsets and expansion of cytotoxic CD4+ T cells in the lungs in severe COVID-19". Clinical Immunology (Orlando, Fla.). 237: 108991. doi:10.1016/j.clim.2022.108991. ISSN 1521-7035. PMC 8961941. PMID 35364330.
- ^ Kaneko, Naoki; Kuo, Hsiao-Hsuan; Boucau, Julie; Farmer, Jocelyn R.; Allard-Chamard, Hugues; Mahajan, Vinay S.; Piechocka-Trocha, Alicja; Lefteri, Kristina; Osborn, Matthew; Bals, Julia; Bartsch, Yannic C.; Bonheur, Nathalie; Caradonna, Timothy M.; Chevalier, Josh; Chowdhury, Fatema (2020-10-01). "Loss of Bcl-6-Expressing T Follicular Helper Cells and Germinal Centers in COVID-19". Cell. 183 (1): 143–157.e13. doi:10.1016/j.cell.2020.08.025. ISSN 1097-4172. PMC 7437499. PMID 32877699.
- ^ a b Semeano, Ana T.; Tofoli, Fabiano A.; Corrêa-Velloso, Juliana C.; de Jesus Santos, Ana P.; Oliveira-Giacomelli, Ágatha; Cardoso, Rafaela R.; Pessoa, Mateus A.; da Rocha, Edroaldo Lummertz; Ribeiro, Gustavo; Ferrari, Merari F. R.; Pereira, Lygia V.; Teng, Yang D.; Petri, Denise F. S.; Ulrich, Henning (April 2022). "Effects of Magnetite Nanoparticles and Static Magnetic Field on Neural Differentiation of Pluripotent Stem Cells". Stem Cell Reviews and Reports. 18 (4): 1337–1354. doi:10.1007/s12015-022-10332-0. ISSN 2629-3277. PMID 35325357. S2CID 247678247.
- ^ a b Yi, Shanyong; Chen, Ke; Zhang, Lihua; Shi, Weibo; Zhang, Yaxing; Niu, Shiba; Jia, Miaomiao; Cong, Bin; Li, Yingmin (2019). "Endoplasmic Reticulum Stress Is Involved in Stress-Induced Hypothalamic Neuronal Injury in Rats via the PERK-ATF4-CHOP and IRE1-ASK1-JNK Pathways". Frontiers in Cellular Neuroscience. 13: 190. doi:10.3389/fncel.2019.00190. ISSN 1662-5102. PMC 6509942. PMID 31130849.
- ^ Sze, Chun-I.; Lin, Yung-Chieh; Lin, Yuh-Jyh; Hsieh, Ting-Hui; Kuo, Yu Min; Lin, Chyi-Her (2013). "The role of glucocorticoid receptors in dexamethasone-induced apoptosis of neuroprogenitor cells in the hippocampus of rat pups". Mediators of Inflammation. 2013: 628094. doi:10.1155/2013/628094. ISSN 1466-1861. PMC 3557631. PMID 23401645.
- ^ Wang, Liang-Chao; Huang, Chih-Yuan; Wang, Hao-Kuang; Wu, Ming-Hsiu; Tsai, Kuen-Jer (2012-05-01). "Magnesium sulfate and nimesulide have synergistic effects on rescuing brain damage after transient focal ischemia". Journal of Neurotrauma. 29 (7): 1518–1529. doi:10.1089/neu.2011.2030. ISSN 1557-9042. PMC 3335109. PMID 22332641.